Last week, I had the honor of giving the concluding keynote lecture at the event “Data-driven mechanistic modelling in life sciences”. This follows a trend of being invited to give more and more keynote and plenary lectures at events, for which I am very grateful. Such longer lectures also give me the chance to expand a little bit more on my point-of-view. The focus of this particular workshop is also something I am very keen to promote, since I think that this particular overlap (mechanistic and data-driven modelling) is under-represented in many communities and conferences.
The centrality of this overlap is actually seen even in our group logo (Fig A). a) The fact that it has an open non-black box, represents the fact that we do mechanistic modelling. b) The data-driven aspect of our models is represent by the purple core in the middle, which represents the fact that we always look for core predictions. Core predictions are predictions that are well-determined from the current prior knowledge and data, even when taking all uncertainties in data and prior knowledge into account.
While I personally think that this is the way to work, and while we have a very well-established workflow for how to develop models in this fashion, mechanistic modelling and data-driven modelling are unfortunately often done in two disjoint communities, with too little overlap (Fig B). Mechanistic modelling often results in mere simulation-based results, which have not been validated using independent data, i.e. data that has not been used to train the model. This is often the case for e.g. PDE and agent-based models, but also common in e.g. theoretical ecology, theoretical biology, etc. It was therefore encouraging too see that one of the presentations at the workshop (by Joshua Bull) looked at spatial models, and on how to quantify the comparison between simulations and data also for spatial models. Data-driven modelling is too often interpreted to mean only machine-learning, narrow AI, and other black-box modelling techniques. While these are big and very hyped communities and approaches at the moment, they are not the only techniques that can be used to do data-driven modelling. In other words, while these black-box models include important techniques, which are useful if one has standardized large-scale data, they also have critical short-comings. Black-box models e.g. have big problems incorporating the type of data that is present in most biological papers, including the prior that is knowledge available. For these reasons, explainability and trustworthiness are challenges. I therefore think that hybrid modelling is the way forward (see e.g. this review, and this example). At the conference, there was also an excellent opening keynote of day 2, by Alvaro Köhn-Luque, which showed some additional and interesting examples of hybrid models.
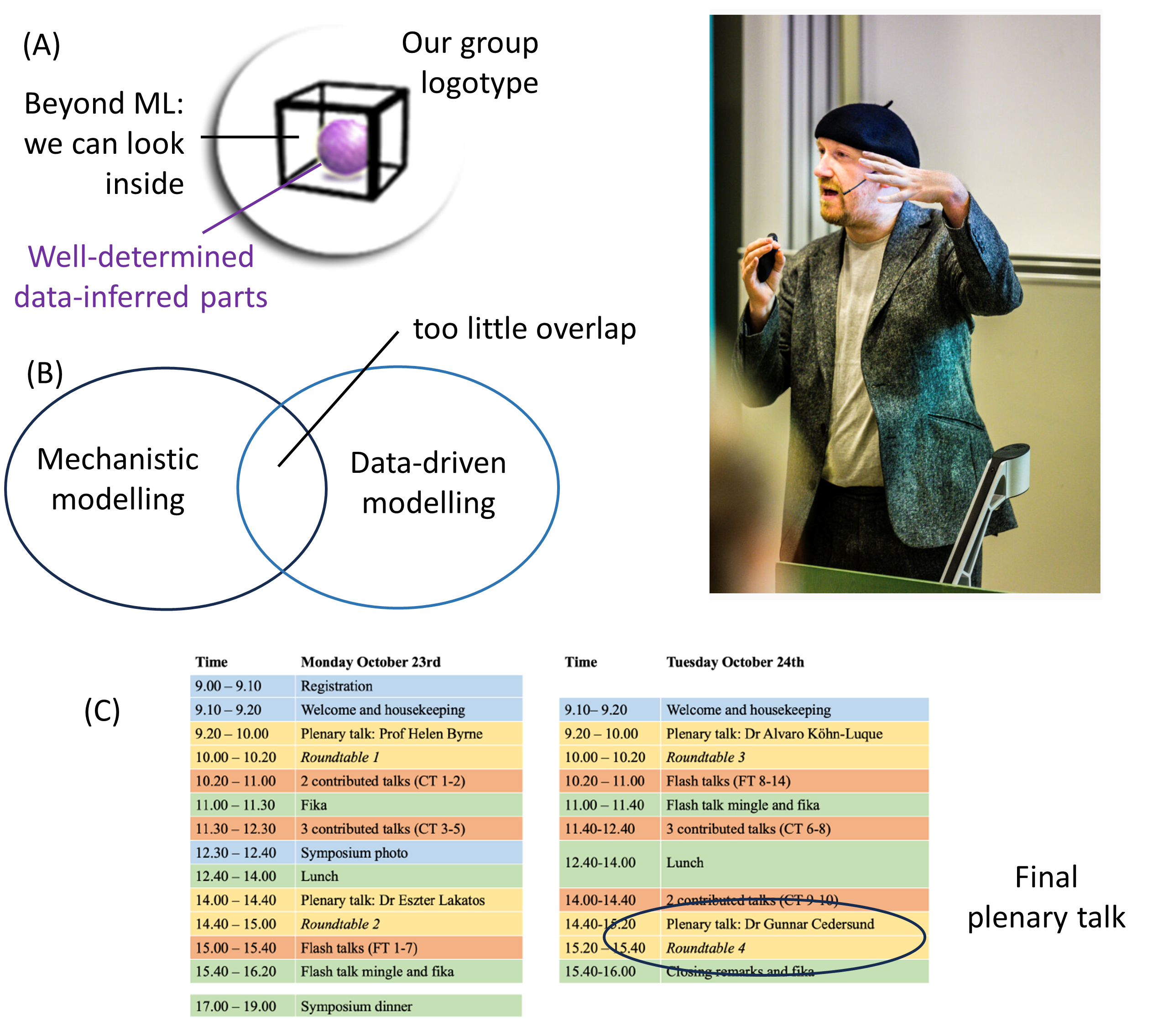
Picture by Thor Balkhed at Linköping University, and taken at another event